The pricing puzzle: Price optimisation for retail
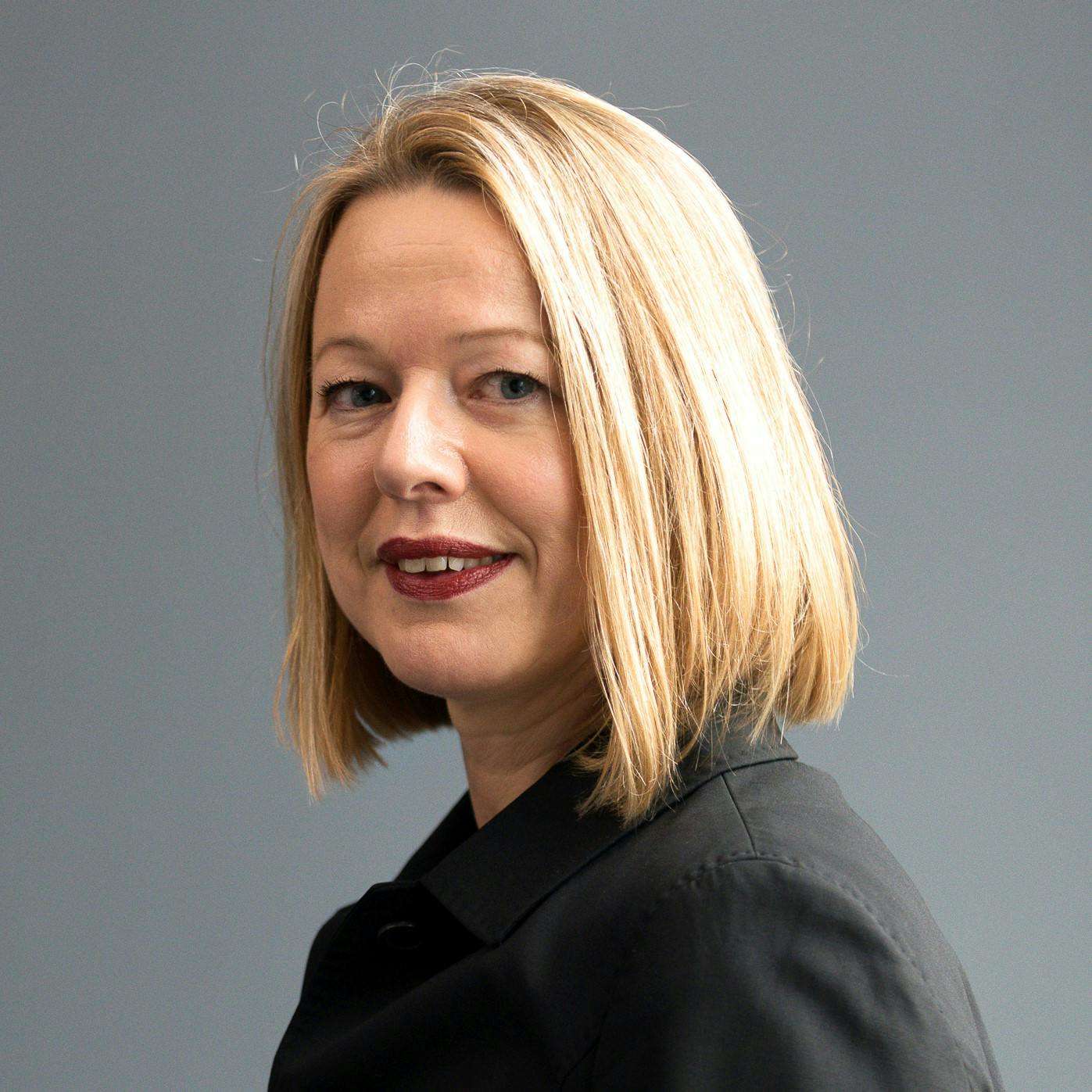
The pricing puzzle: Price optimisation for retail
AI can be used to answer the most important questions in retail - from the strategic to the operational.
Price optimisation is primed for an AI solution.
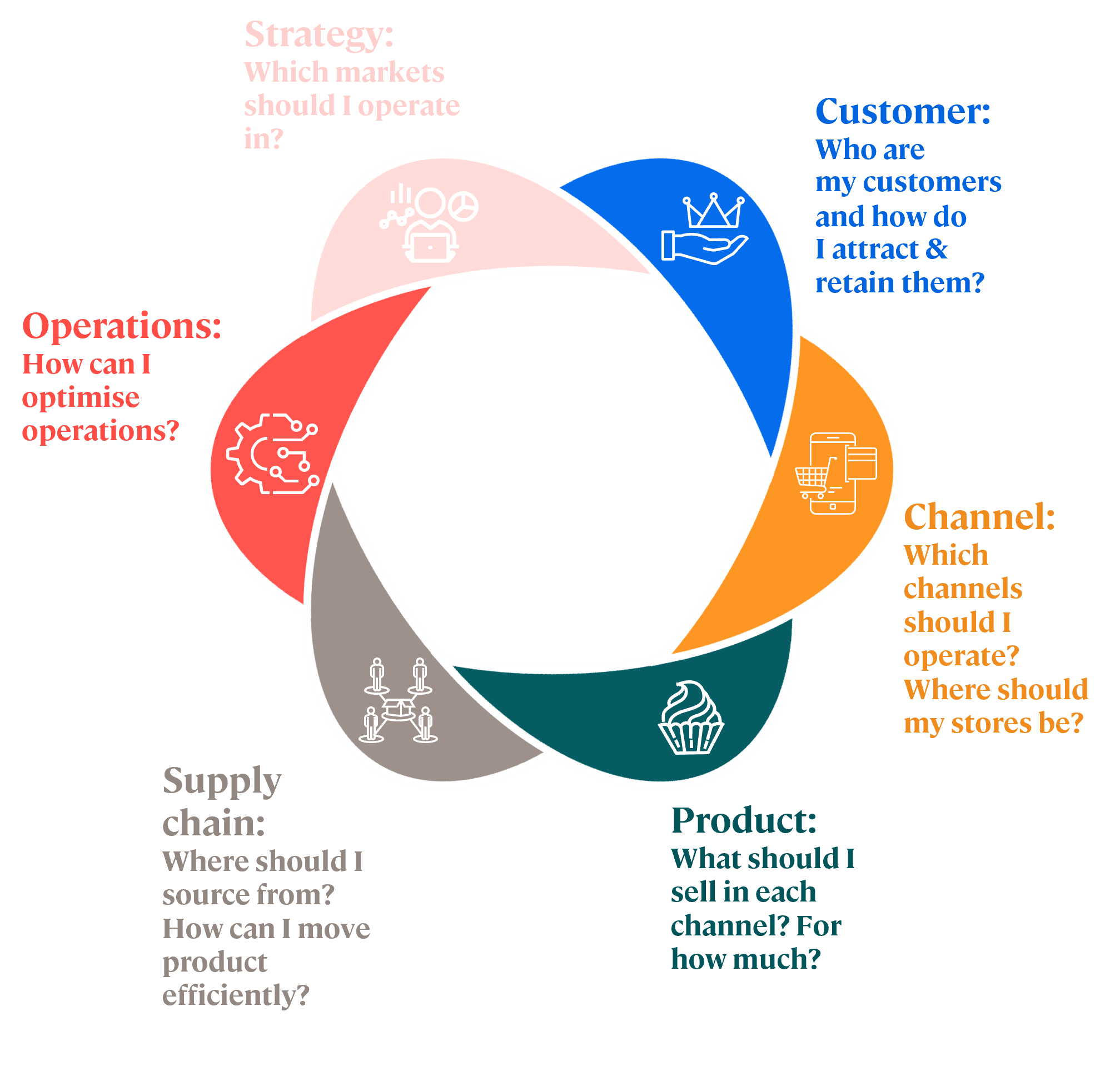
The issue:
The pricing puzzle
UK retailers spend millions of pounds per week on pricing investments. These can be planned promotions partly funded by branded suppliers but are often reactions to competitive pressure from rivals.
Saving time, saving money?
It takes a lot of time and effort to (i) identify which SKUs need careful price management (ii) analyse the level of price change, cost, likely impact on sales, adjusted stock requirements and (iii) implement in stores.
Sub-optimal pricing squeezes margins
Limited capacity to proactively manage prices combined with sales-based incentives can lead to knee-jerk pricing decisions by category managers. New prices may increase sales or basket size temporarily but often cause to longer term margin erosion.
The opportunity
Advanced algorithms can recommend the right pricing trade-off across thousands of SKUs. An AI-based tool can also be linked to competitor’s price point data - usually outsourced to third party specialists who scrape websites, analysing 25-40k SKUs. SKU-level price band recommendation can allow you to perform drill-down analysis and simulate impact of a price change on sales.
The solution: An AI-powered recommendation engine
Build a unique and powerful data asset with multiple uses by combining your data with external sources
Deploy cutting-edge data science methods such as reinforcement learning to predict customer behaviour and improve predictions over time
Create a bespoke solution tailored to your specific requirements
Understand price elasticity, predict customers' willingness to pay, optimise pricing, forecast impact of promotions, analyse live market dynamics, compare with competitors.
The Arca Blanca approach:
Size optimisation powered by data science
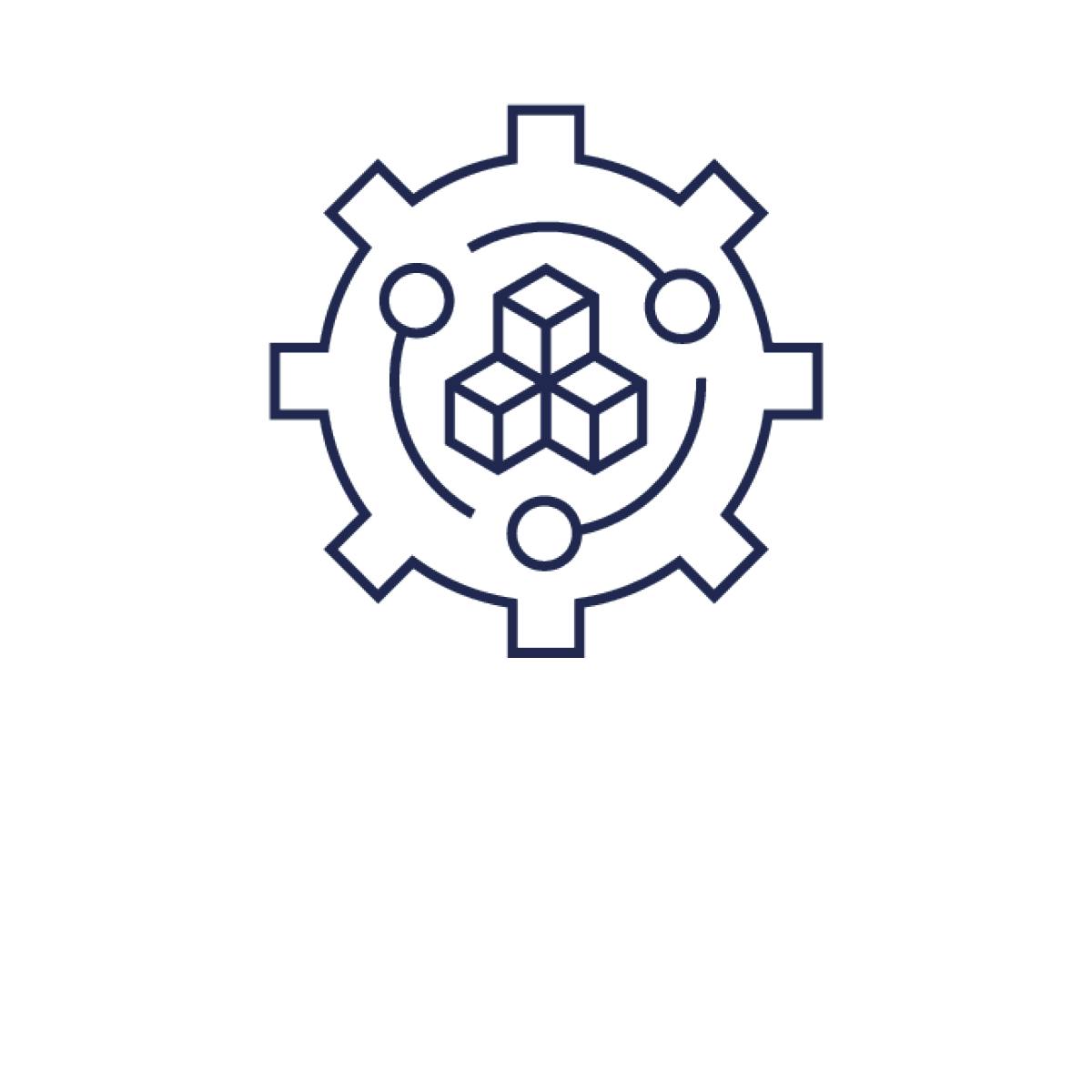
1) Data preparation
Establish data quality and availability for the key parameters by store and channel, including full-price sales, discounted prices sales, stock availability, commitments, SKU hierarchy, and attributes.
2) Data modelling
Develop two data science models to (i) estimate SKU price elasticity and forecast sales at a given price, and (ii) estimate cost of a price change.
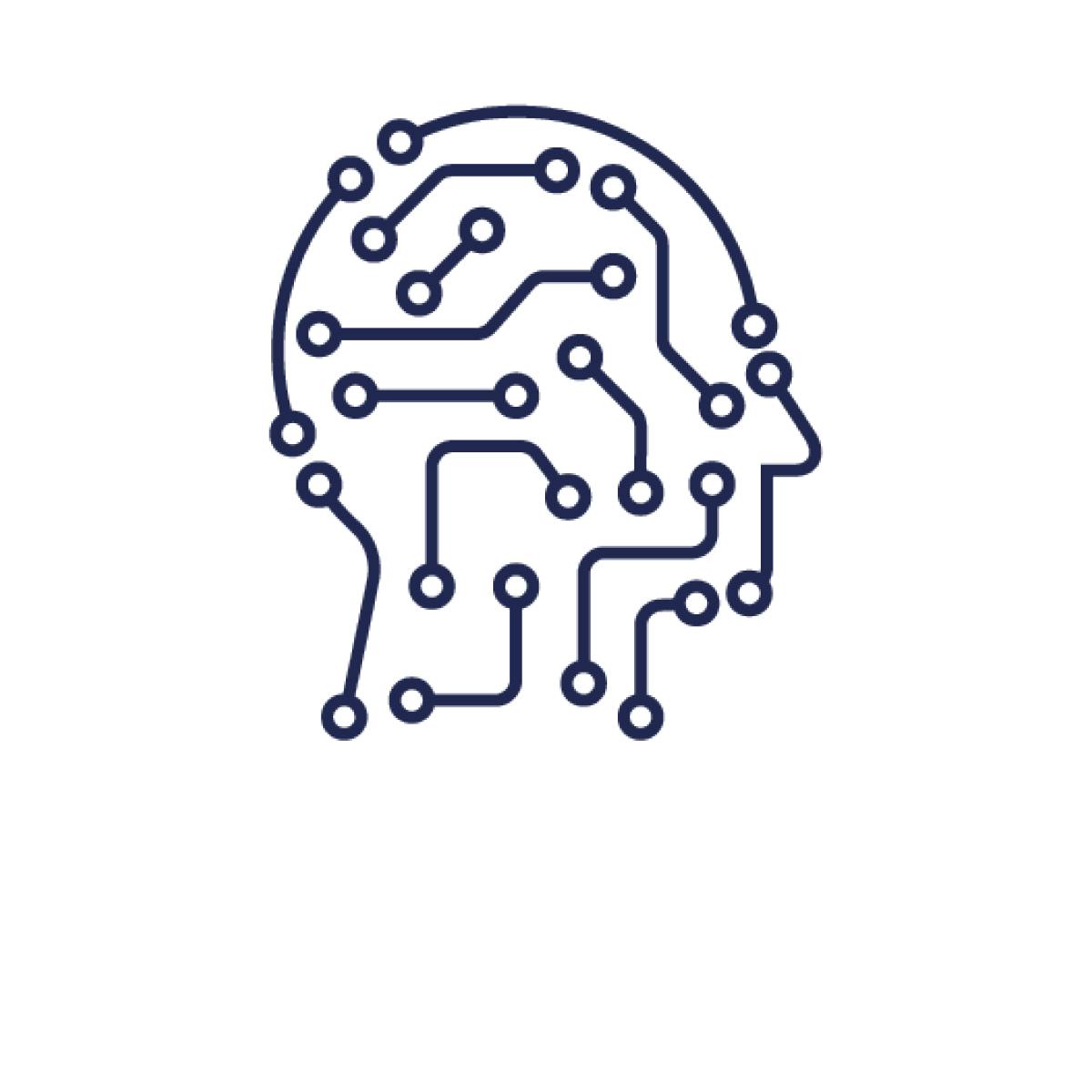
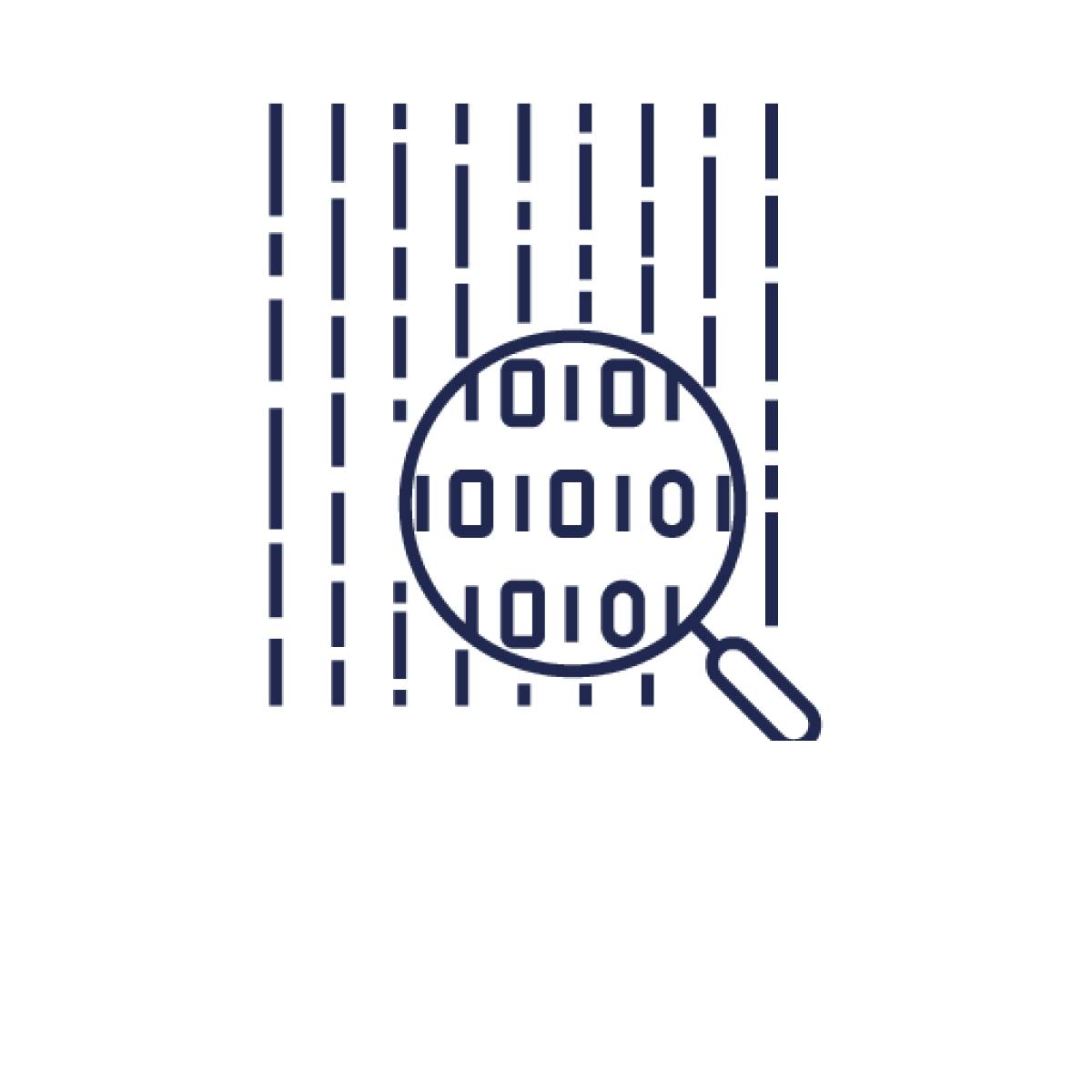
3) Third party data
Establish link with 3rd party price comparison service providers to determine how a given SKU is performing against competitors.
4) Price recommendations
Use data science to determine the optimum pricing bands based upon competitor pricing, price elasticity, and cost of price change
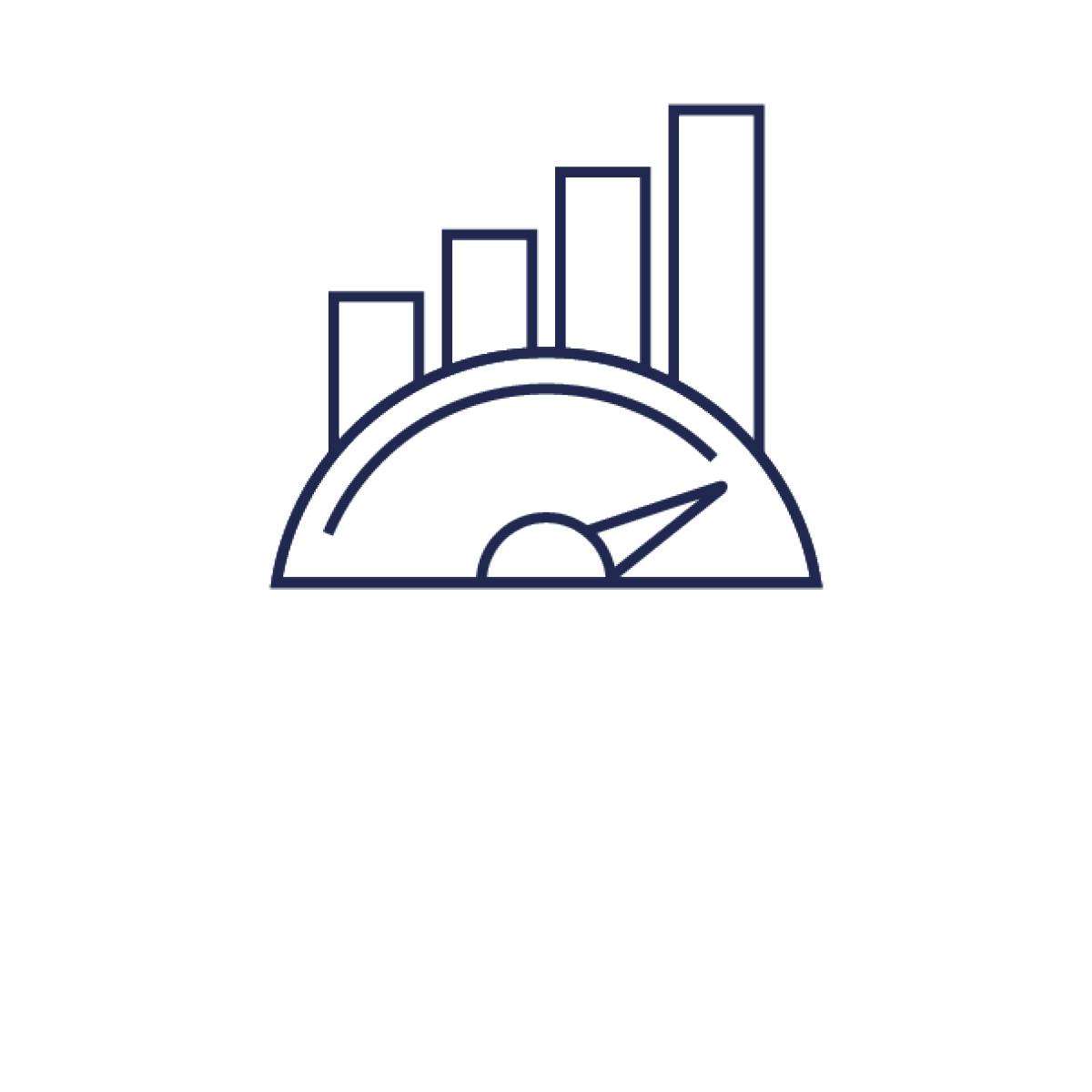
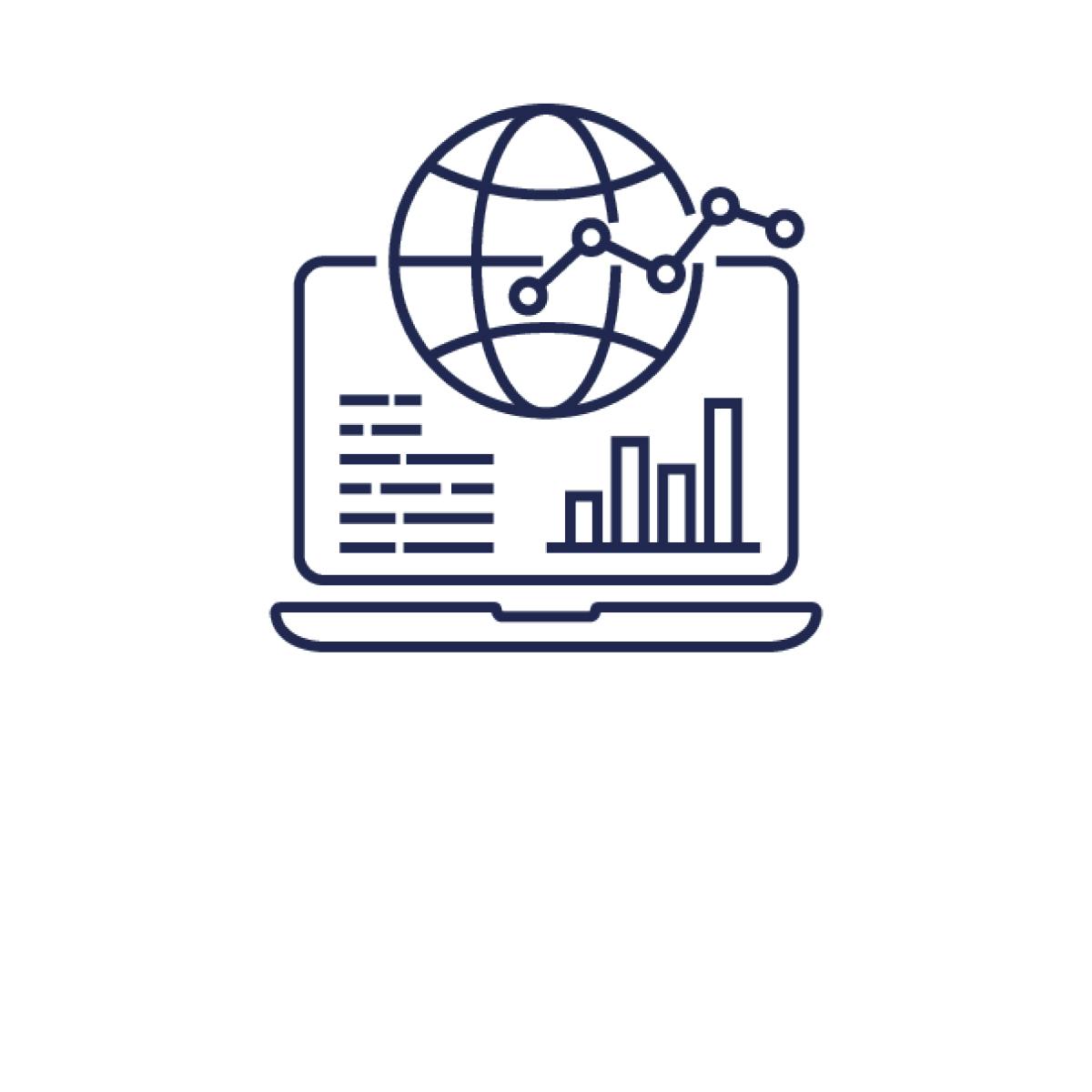
5) Sticker-price interface
Integrate the automated pricing system with store’s sticker labelling system in order to realise pricing value without impacting operational efficiency within stores.